Autism spectrum disorder ASD affects a person’s cognitive, verbal, object recognition, communication, and social capacities. Early diagnosis of ASD can help diagnose and mitigate its effects. AI can detect ASD earlier than traditional methods. This study aimed to develop a machine learning model to better identify ASD in different age groups. We employed feature selection algorithms on ASD datasets from toddlers, children, adolescents, and adults. Different classifiers were then applied to these datasets and evaluated using prediction accuracy, kappa statistics, the f1-measure, and AUROC. We also assessed classifier performance using a non-parametric test. For the toddler, child, adolescent, and adult datasets, Support Vector Machine (SVM) performed better than other classifiers, achieving 97.82 percent accuracy for the RIPPER-based toddler subset, 99.61 percent accuracy for the CFS and BIC-based child subset, 95.87 percent accuracy for the Boruta-based adolescent subset, and 96.82 percent accuracy for the CFS-based adult subset. Then, we applied the Shapley Additive Explanations (SHAP) approach to feature subsets with the best accuracy and ordered their features.
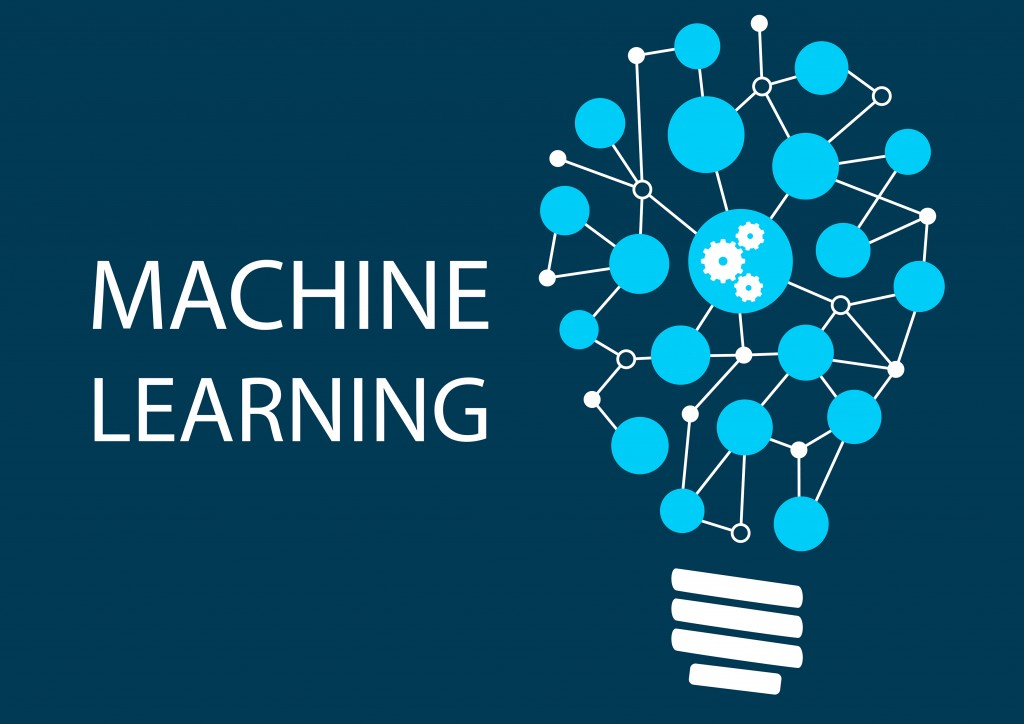
Machine Learning Models for Early Stage Detection of ASD
More from Cyber SecurityMore posts in Cyber Security »
More from Featured PostsMore posts in Featured Posts »
More from latest_postsMore posts in latest_posts »
Be First to Comment